When it Comes to Optimizing Wind Energy Production, Nature is the Best Teacher
The technology draws from the laws and physics of a natural swarm, i.e., Starling Murmuration, where optimization aims to treat individual turbines on farms as a collective and cooperative ‘swarm’ (as opposed to approaching optimization on a turbine-by-turbine basis). By adopting this new approach to optimizing wind assets, wind energy stakeholders stand to benefit from a 3-5% year on year improvement in AEP, helping the wind industry rise to the challenge of global net zero by 2050.
February 14, 2022. By News Bureau

Widespread underperformance of wind farms is a growing issue for the wind energy sector. But as expectations for the industry increase as part of the energy transition, operators are looking to ensure consistent revenue growth to attract further investment and justify increasing government support.
Underperformance and low profit margins pose serious financial implications – and opportunity costs – for wind farm owners, suppliers and investors.
However, existing solutions to improve Annual Energy Production (AEP) of wind farms to help plants meet performance projections are failing to deliver or incur significant expenditure on repowering, blade replacement and other hardware upgrades, leaving wind operators without clear options in the race to improve revenues.
Underperformance and low profit margins pose serious financial implications – and opportunity costs – for wind farm owners, suppliers and investors.
However, existing solutions to improve Annual Energy Production (AEP) of wind farms to help plants meet performance projections are failing to deliver or incur significant expenditure on repowering, blade replacement and other hardware upgrades, leaving wind operators without clear options in the race to improve revenues.
In response to these growing pressures, WindESCo has developed a new, readily deployable AEP optimization technology that applies lessons learnt from nature is helping owners, suppliers and investors increase Return on Investment (ROI).
The technology draws from the laws and physics of a natural swarm, i.e., Starling Murmuration, where optimization aims to treat individual turbines on farms as a collective and cooperative ‘swarm’ (as opposed to approaching optimization on a turbine-by-turbine basis).
By adopting this new approach to optimizing wind assets, wind energy stakeholders stand to benefit from a 3-5% year on year improvement in AEP, helping the wind industry rise to the challenge of global net zero by 2050.
The wind industry’s underperformance problem
One of the biggest challenges of wind energy production is accelerating the AEP of wind turbines and wind projects. This is especially difficult when plants fail to meet their performance projections when year-on-year profit margins are squeezed under an increasingly competitive market.
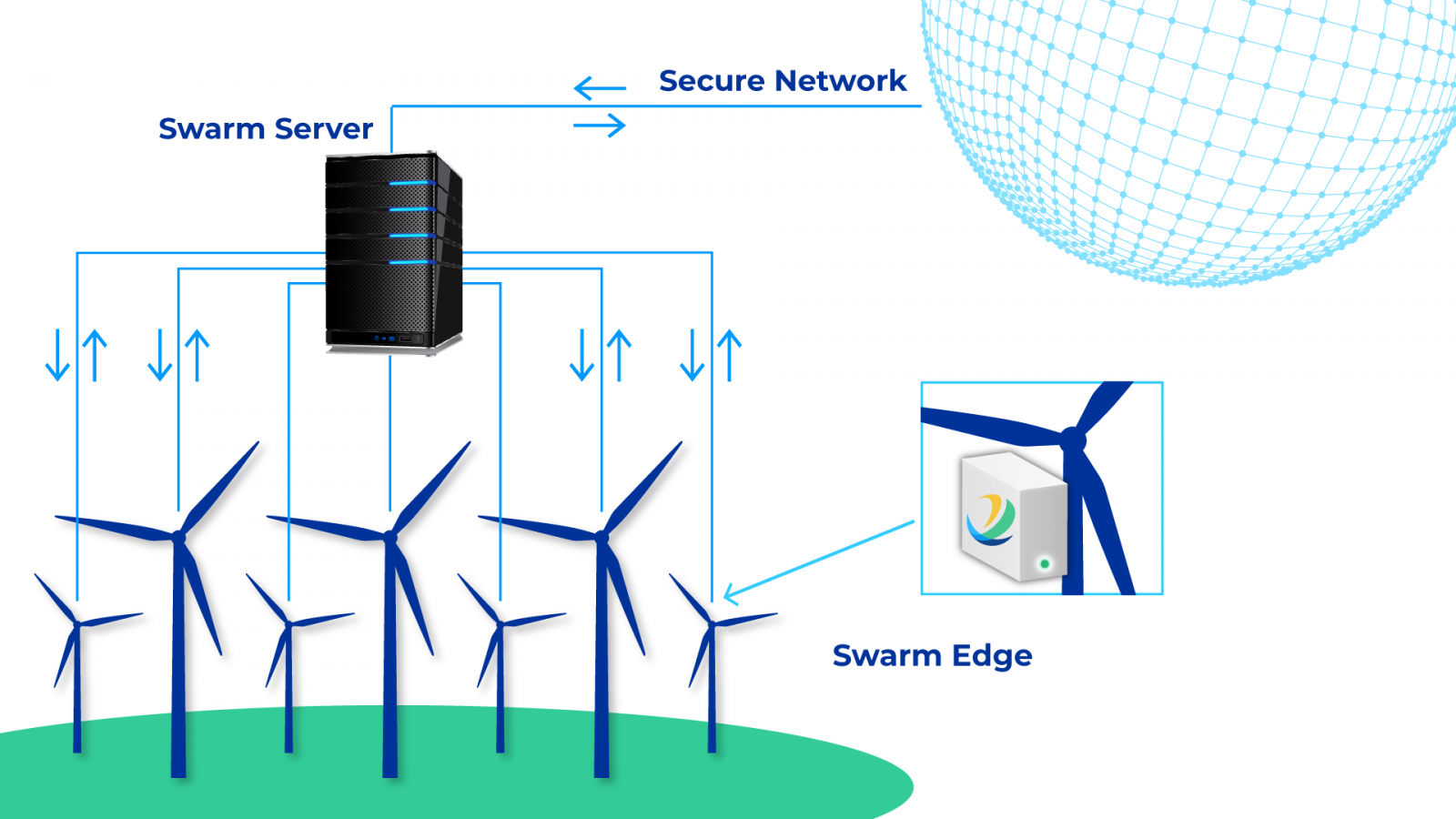
Inaccurate pre-construction models of plants have previously resulted in significant wake loss, further amplifying the underperformance challenge. The wake generated from individual turbines can disrupt the effectiveness of adjacent turbines, decreasing overall site performance. Research by the National Renewable Energy Laboratory (NREL) shows that sites lose between 10-15% of production as a result of inaccurate pre-construction models and turbine-to-turbine wake effects.
Current AEP improvement solutions mostly center on Asset Performance Monitoring (APM) on a turbine-by-turbine basis. Operators employ control strategies designed to maximize individual asset production, rather than approaching plants as collective and cooperative units. This isolated operational approach reinforces reactive and localized turbine control behavior and increases turbine-to-turbine wake effects, which limit plant optimization and accelerate asset aging and degradation.
Underperformance is a widespread industry problem. However, current solutions that approach AEP optimization on an individual, turbine-by-turbine basis don’t go far enough to increase ROI for wind investors in an increasingly challenging market. The result is that millions of dollars are being invested in renewable APM systems that fail to deliver consistent AEP improvement.
The solution: Holistic plant optimization and control
Fortunately, a novel and readily deployable technology is enabling wind farm operators to adopt a new approach to AEP optimization, providing significant ROI for wind sector stakeholders.
Inspired by natural swarming behavior, the new technique of optimization looks at a wind site as a single system, rather than individual turbines. It enables turbines to become socially interactive with one another, reacting to neighboring units and continuously sharing information. Swarming, as the technology’s name suggests, allows turbines to communicate their direction, strength, and any changes in wind resource, and shift each turbine’s operational profile to optimize energy production across the plant as whole.
The technology combines smart sensors and analytics into a complete AEP improvement package. It deploys edge servers on each wind turbine, which are first connected to a site server for control and then to a secured cloud server. It includes a series of analytics applications including Yaw by Consensus that work together to optimize AEP and measure gains across the site. Measurement is done against a control group of turbines following a DNV-approved methodology and Independent Engineering (IE) oversight.
To deliver the most benefit, the implementation of the technology should be conducted on a site-by-site basis. This enables turbines to characterize local variability to optimize decisions around yaw alignment, balancing increased production with turbine lifespan.
Swarming leverages a proven algorithm to correct yaw misalignment prior to incurring energy loss and allows for variations in yaw error as a result of local wind differences. It opens communication between turbines and uses operational data to off-yaw select turbines, reducing wake interferences between turbines.
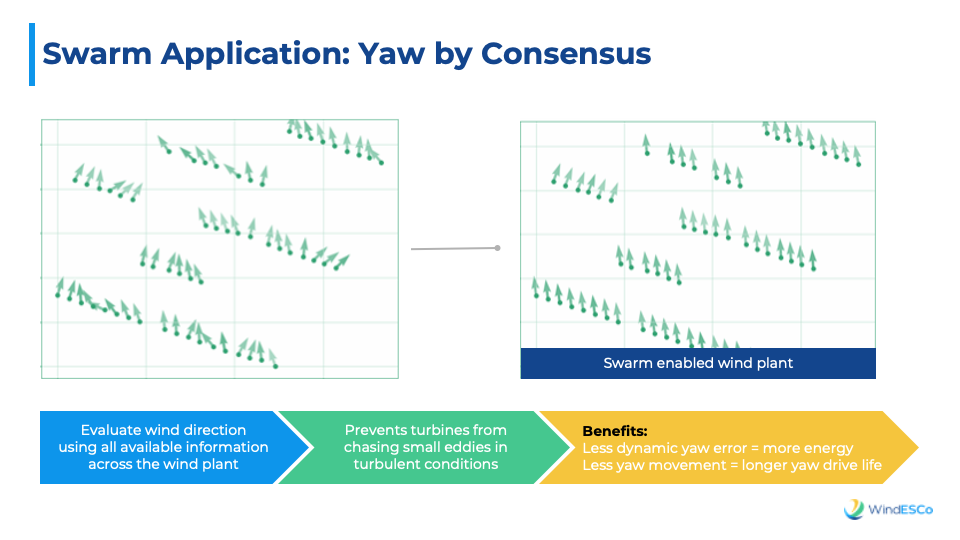
The benefits to wind industry stakeholders
The Swarming technology was tested on a wind farm in north-eastern US to demonstrate its ability to increase asset longevity and establish the financial case for implementation.
Analysis from the testing showed an anticipated change of less than 1% in fatigue loading on turbines. Experimental results for the period analyzed supported this result, showing no statistically significant difference in the fatigue loading on the turbines when wake steering. These results are directly transferable to commercial installations and ensure the delivery of long-term value over the lifecycle of a wind farm.
The testing outcomes deliver direct benefits for asset managers, fund manager and Original Equipment Manufacturers (OEMs). Asset managers realize a 3-5% AEP improvement across an entire fleet, with cumulative improvements stacking over longer periods of time to unlock a continuous trend of performance gains.
For fund managers, the continuous 3-5% AEP improvements accrue a substantial ROI on existing installations, enabling legacy assets to compete with newer, more technologically advanced assets.
For OEMs, the technology means they can provide their customers with certainty and security on the performance profile of their components and how to leverage their Full-Service Agreements and LongTerm Service Agreements to accelerate AEP on a fleet-wide basis.
Nature is our greatest teacher
The wind industry has the opportunity to increase profit margins without huge capital investment. First, it needs to rethink its approach to AEP improvement, and unlock the data and revenue already in its assets.
Operators can learn a lot from collective and cooperative examples seen in nature, such as the starling swarm. By adopting readily deployable new technology and applying the same idea to assets, wind farm owners and operators will increase AEP– and subsequently their overall ROI.
- Ed Wagner, Chief Revenue Officer, WindESCo
If you want to cooperate with us and would like to reuse some of our content,
please contact: contact@energetica-india.net.
please contact: contact@energetica-india.net.